
“Attention Net didn’t sound very exciting,” said Vaswani, who started working with neural nets in 2011. How Transformers Got Their NameĪttention is so key to transformers the Google researchers almost used the term as the name for their 2017 model. “Now we see self-attention is a powerful, flexible tool for learning,” he added. “Machine translation was a good vehicle to validate self-attention because you needed short- and long-distance relationships among words,” said Vaswani. “Meaning is a result of relationships between things, and self-attention is a general way of learning relationships,” said Ashish Vaswani, a former senior staff research scientist at Google Brain who led work on the seminal 2017 paper. She poured water from the pitcher to the cup until it was empty. We know “it” refers to the cup, while in the sentence: She poured water from the pitcher to the cup until it was full. With these tools, computers can see the same patterns humans see. Attention units follow these tags, calculating a kind of algebraic map of how each element relates to the others.Īttention queries are typically executed in parallel by calculating a matrix of equations in what’s called multi-headed attention. Transformers use positional encoders to tag data elements coming in and out of the network. A look under the hood from a presentation by Aidan Gomez, one of eight co-authors of the 2017 paper that defined transformers. Small but strategic additions to these blocks (shown in the diagram below) make transformers uniquely powerful. Like most neural networks, transformer models are basically large encoder/decoder blocks that process data. Transformers now dominate popular performance leaderboards like SuperGLUE, a benchmark developed in 2019 for language-processing systems.
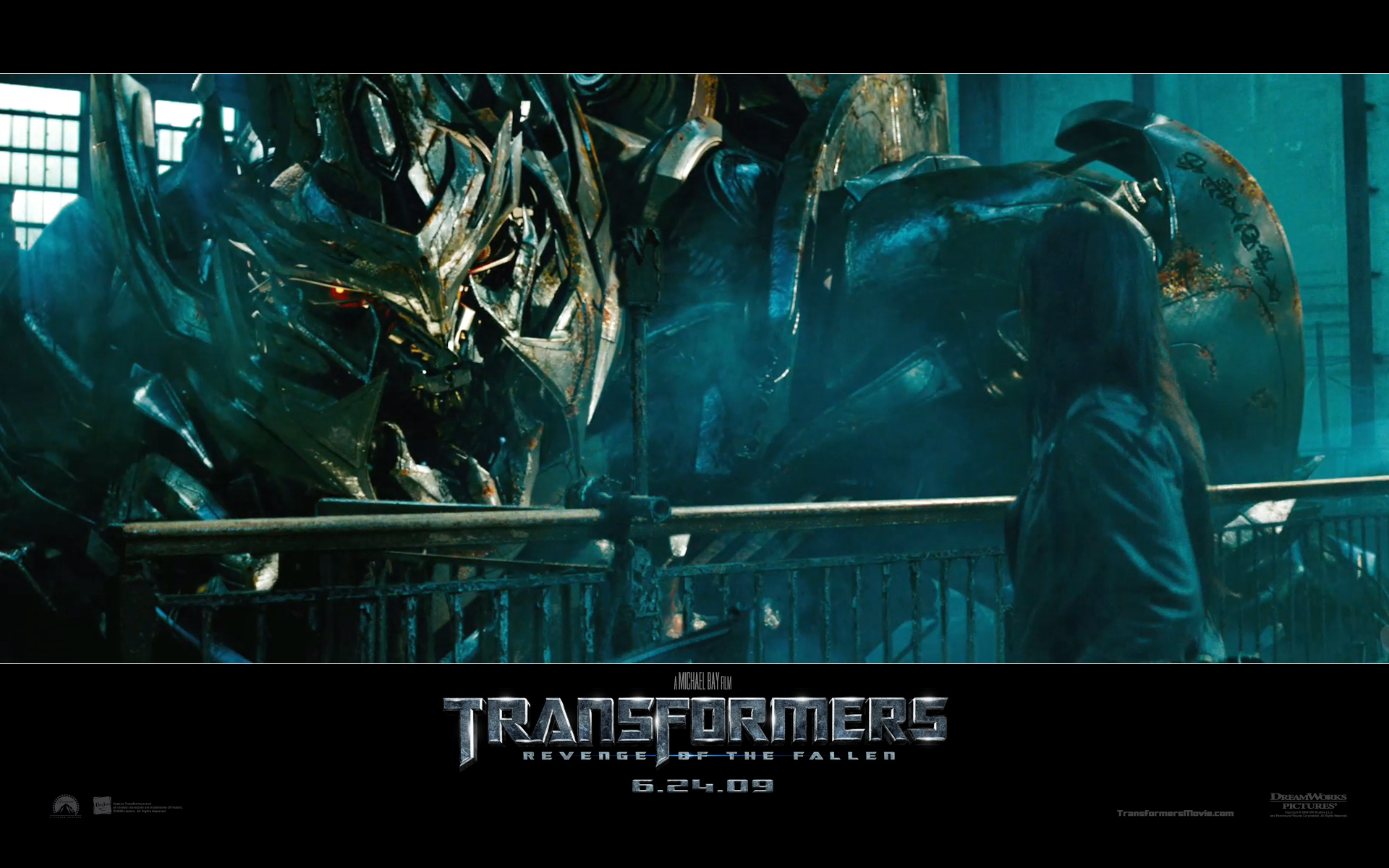
In addition, the math that transformers use lends itself to parallel processing, so these models can run fast. By finding patterns between elements mathematically, transformers eliminate that need, making available the trillions of images and petabytes of text data on the web and in corporate databases. No Labels, More Performanceīefore transformers arrived, users had to train neural networks with large, labeled datasets that were costly and time-consuming to produce. That’s a radical shift from a 2017 IEEE study that reported RNNs and CNNs were the most popular models for pattern recognition. Indeed, 70 percent of arXiv papers on AI posted in the last two years mention transformers. Transformers are in many cases replacing convolutional and recurrent neural networks (CNNs and RNNs), the most popular types of deep learning models just five years ago. “Transformers made self-supervised learning possible, and AI jumped to warp speed,” said NVIDIA founder and CEO Jensen Huang in his keynote address this week at GTC. Stanford researchers say transformers mark the next stage of AI’s development, what some call the era of transformer AI. Created with large datasets, transformers make accurate predictions that drive their wider use, generating more data that can be used to create even better models. That enables these models to ride a virtuous cycle in transformer AI. The Virtuous Cycle of Transformer AIĪny application using sequential text, image or video data is a candidate for transformer models. People use transformers every time they search on Google or Microsoft Bing. Transformers can detect trends and anomalies to prevent fraud, streamline manufacturing, make online recommendations or improve healthcare. Transformers, sometimes called foundation models, are already being used with many data sources for a host of applications. They’re helping researchers understand the chains of genes in DNA and amino acids in proteins in ways that can speed drug design. Transformers are translating text and speech in near real-time, opening meetings and classrooms to diverse and hearing-impaired attendees. The “sheer scale and scope of foundation models over the last few years have stretched our imagination of what is possible,” they wrote. Stanford researchers called transformers “foundation models” in an August 2021 paper because they see them driving a paradigm shift in AI. They’re driving a wave of advances in machine learning some have dubbed transformer AI.
#TRANSFORMERS ROBOTIZE ME SERIES#
Transformer models apply an evolving set of mathematical techniques, called attention or self-attention, to detect subtle ways even distant data elements in a series influence and depend on each other.įirst described in a 2017 paper from Google, transformers are among the newest and one of the most powerful classes of models invented to date.
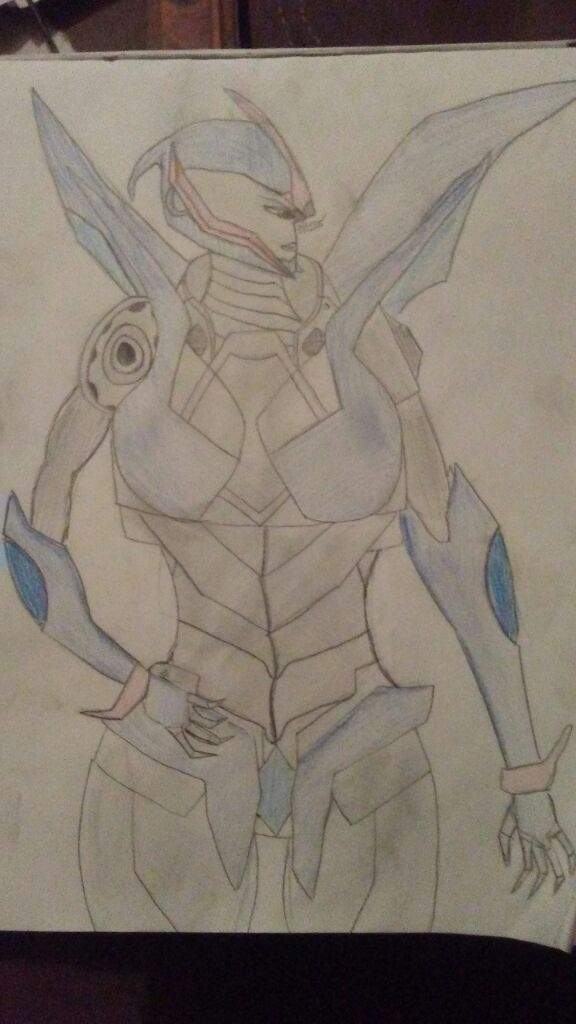
So, What’s a Transformer Model?Ī transformer model is a neural network that learns context and thus meaning by tracking relationships in sequential data like the words in this sentence.
#TRANSFORMERS ROBOTIZE ME TV#
They’re not the shape-shifting toy robots on TV or the trash-can-sized tubs on telephone poles. If you want to ride the next big wave in AI, grab a transformer.
